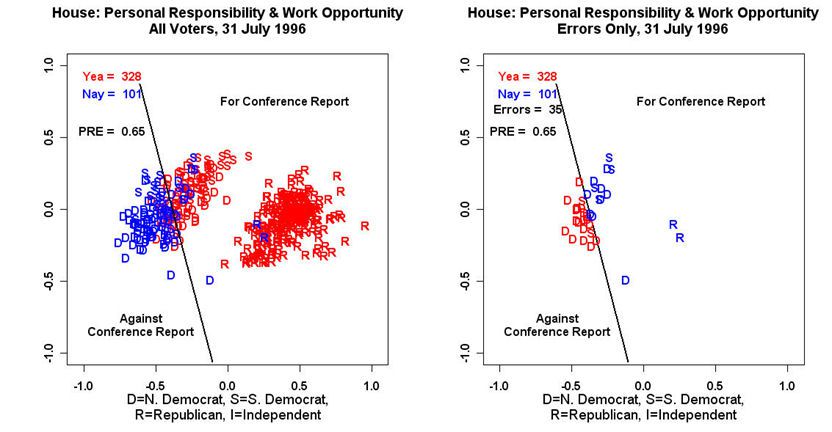
Suppose you want to know how conservative or liberal your Congressional representative is. Is there a way to do this that doesn’t involve sorting through a small mountain of votes and making an educated guess?
Political scientists have been working on this problem for decades, and have largely solved it. They can take a legislator’s voting record and use it to produce a mathematical estimate of that legislator’s ideology compared to his peers in Congress. And it’s not just votes – they can make similar estimates with campaign contributions, surveys, speeches, and even Twitter followers.
While the particular method political scientists use to make these estimates has varied over the years, conceptually all of them are variations of a technique called factor analysis.
Researchers employ factor analysis to simplify data. In this example, legislators may vote on 500 bills in a session. But to be familiar with our congressperson, we don’t have to keep track of their votes on all 500 bills – if we know his or her political leanings, specifically if the congressperson is conservative or liberal, we can predict many of their votes from that fact alone. Just one number – one “factor” – representing political ideology can summarize the data contained in all 500 votes. Factor analysis looks for these kinds of patterns that allow us to summarize larger sets of data with just a few numbers.
Political scientists weren’t the first to do this kind of analysis. Early models were inspired by work in psychology.
Which brings us to the mini-debate that has sprung up, with Sam Harris and Charles Murray on one side, and PZ Myers and Cosma Shalizi (indirectly) on the other, about IQ.
Like Myers, I’m not a fan of Sam Harris and I refuse to listen to his monotone for 2 hours. And I agree with the Eyes On The Right post that Myers quotes. Where I, at long last, finally disagree is when he dismisses the concept of IQ altogether, citing an article by Cosma Shalizi (in the comments).
Shalizi’s article – read it here – attempts to undermine factor analysis as a whole, as well as it’s specific application to the concept of g, or general intelligence (g is what IQ is supposed to measure). The main workhorses of his argument are two mathematical examples. But of the two, the first one is incorrect and the second one doesn’t prove what he claims.
The first example accidentally assumes what it sets out to prove. Shalizi generated the numbers in his matrix, which represent correlations between test scores, by choosing numbers at random between zero and one. I scratched my head when reading this part. Typically a person running a simulation would generate test scores first, and calculate the correlations directly from the scores. Instead Shalizi first generates the correlations between test scores, then creates the test scores to match the correlations.
This backwardness allows Shalizi to make a mistake he shouldn’t have made. Test scores that aren’t influenced by a common factor should have correlations clustered around zero – that is, the score on one test shouldn’t predict the scores on other tests. Shalizi, however, chooses the correlations by randomly choosing between zero and one, in effect assuming that many of the tests are correlated with each other. The factor analysis then finds a factor in this case because the numbers really do indicate an underlying factor. If Shalizi started by generating factor-less test scores, and calculated the correlations from those test scores (as opposed to randomly generating the correlations), he would quickly discover that this example doesn’t work. Factor analysis may erroneously discover a factor when applied to small amounts of data, but for larger data sets it doesn’t return spurious false positives like this. That’s just not how it works.
The second example is technically correct, but the implications are milder than he suggests. Yes, as demonstrated, IQ may be a summation of many smaller factors, but that doesn’t imply that it’s not a real or useful concept. The NOMINATE ideology scores used by political scientists are also based on many small factors – votes on issues ranging from abortion to taxes to NATO. Yet we all know the difference between conservative and liberal, and given a placement along the left-right axis we can make decent predictions about a person’s views on any number of issues. Clearly the left/right distinction in American politics is not a figment of our imagination, or a statistical artifact, even though it combines many smaller issues into one category. Likewise, though IQ may be the result of many smaller factors (and genetically speaking, I imagine it is), that doesn’t imply it’s not real. It’s correlated – not perfectly correlated, but correlated – with a variety of things, like knowing what the word “vicarious” means, solving MENSA puzzles, and finishing college. These correlations just happen to match one definition of the word intelligence, in the same way that factor analysis of rollcall votes leads to scores that match our understanding of the liberal-conservative spectrum in politics.
Political scientists debate the biases in scores generated by political factor analysis techniques, but there’s basically no debate that the left-right political spectrum is a real thing, and these scores have served as the backbone for a huge amount of research in the last 30 years. As far as I can tell g is in the same place.
My expertise is limited to statistics and social science, so I won’t comment on the debate about to what extent IQ is determined by genes. And I’m skeptical, to put it mildly, of Charles Murray’s policy views. But factor analysis is real.
Disclaimer: Yes, I did read The Bell Curve, about 10 years ago. I think it’s got some rigorous arguments mixed with some really weird arguments. And no, I didn’t encounter any censorship: I rented the book from my local library with no mishap.
Advertisements Share this: